Salesforce Data Cloud—a Customer Data Platform (CDP)—helps build a complete 360-degree view of your customers.
It brings together different data sources that track customer interactions across your business, creating one complete, unified customer profile to deliver better experiences and insights. You can also add extra information, like store details (location, type, region etc.), to make your customer profiles even more complete and useful for deeper segmentation and analysis.
Data Cloud’s key advantage is data harmonization. It focuses on bringing different data sources together and organizing them in a way that makes sense for your business.
Note: Salesforce Data Cloud isn’t designed to replace your data warehouse. Not every dataset belongs here, and specialized platforms exist for complex storage, transformations, and third-party integrations. Be selective about what you ingest—unnecessary data and jobs create system load, slow down processing, and potentially increase costs depending on your contract terms.
Let’s explore the key concepts and practical steps required to successfully bring your data into Data Cloud. In this guide, we’ll cover the essentials you need to know for effective data ingestion.
Key terms and concepts
Before we proceed, let’s clarify the key terminology and theoretical concepts you’ll encounter when working with Data Cloud.
The data in Salesforce Data Cloud follows a specific path:
Data Stream Objects (DSO) → Data Lake Objects (DLO) → Data Model Objects (DMO)
Most data you import begins as a DSO. However, some data sources skip the DSO stage and appear directly as DLOs in the data lake.
Think of this like rivers and lakes in nature: streams (DSOs) can flow into a lake (DLO). And just as some natural lakes have no rivers feeding them but are filled by underground springs or rainfall, some DLOs exist without corresponding DSOs.
Data Model Objects (DMOs) are the business-ready versions of your data that have been processed and structured for specific use cases, You get them available after you map DLOs to DMOs.
You’ll also see the term feed throughout this article. While it’s not official Salesforce terminology, it’s commonly used to describe regularly updated data flows. We’ll use this term to refer to files that continuously move into your system rather than one-time uploads. For example, daily order exports, customer activity logs, or campaign performance data that continually update your Data Cloud environment.
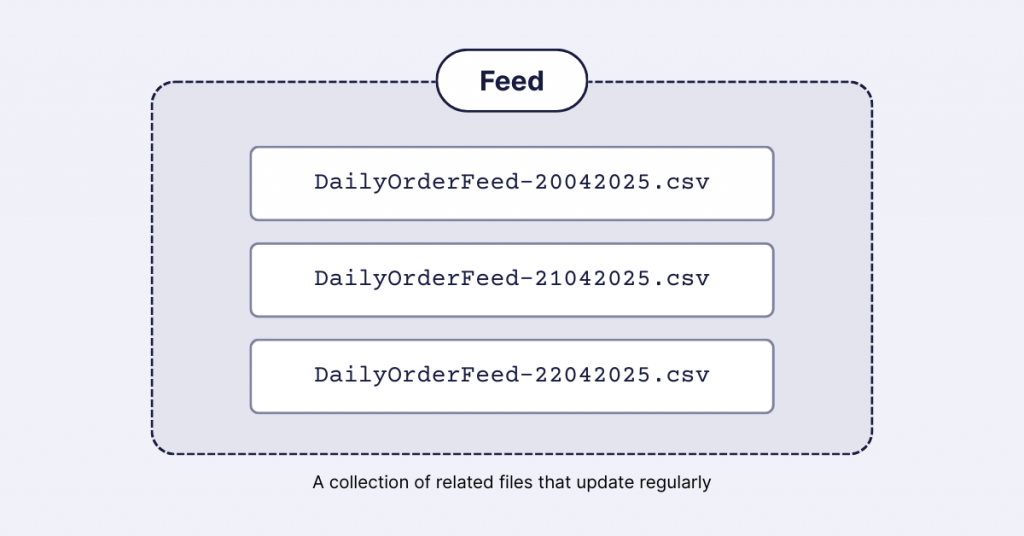
Choosing and setting up your data source connector
When creating a new data stream, start by clicking “New” on the data stream page:

Next, you’ll need to select your data source. Data Cloud offers numerous connector options (the image below shows just a preview of the available choices):
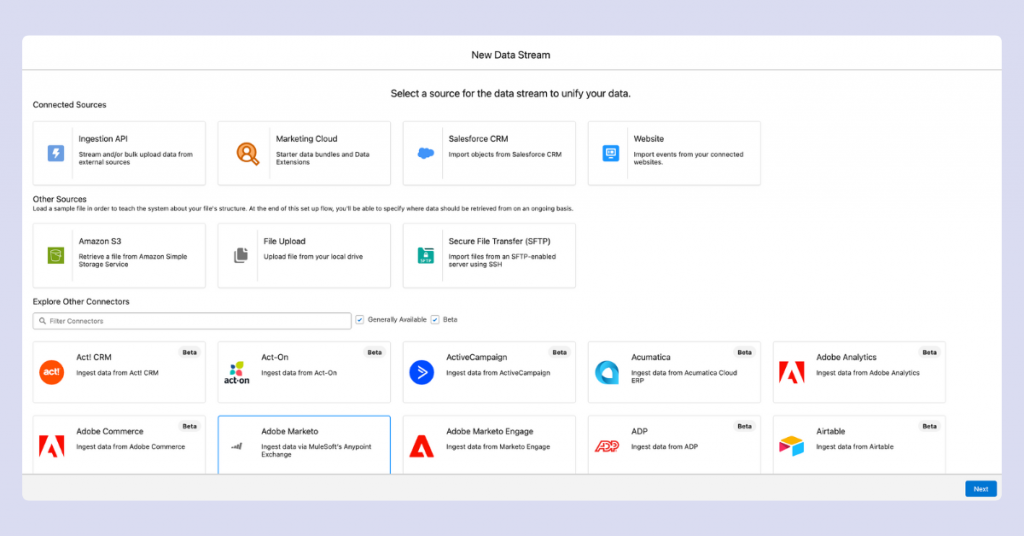
The most commonly used connectors include:
- Other Salesforce systems (Marketing Cloud, CRM)
- Cloud data storage platforms (AWS S3, etc.)
- SFTP servers
If you need to connect different Salesforce systems or streamline data management across platforms, our Salesforce services help you set up, optimize, and scale your Salesforce ecosystem.
While file uploads work for smaller datasets, it’s generally better to use a central data storage system like S3 or Redshift as your connector. This approach prevents data fragmentation and promotes better data accessibility across your organization.
To set up these connections:
1. Navigate to Setup → Platform tools → Data Cloud → Other Connectors
2. Select your desired connector (in this example, S3):
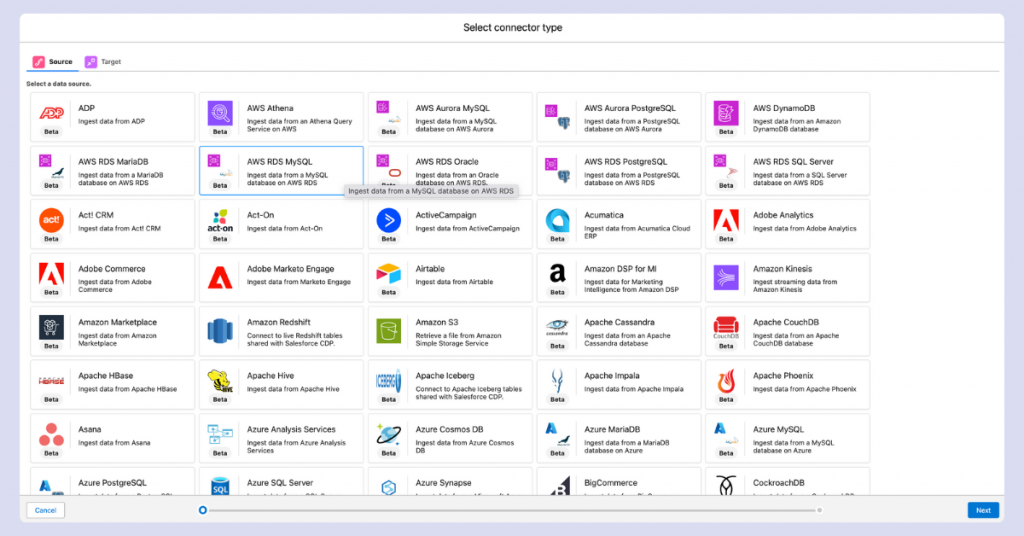
3. Enter the necessary access details and use the “Test Connection” button to verify that Data Cloud can access your data
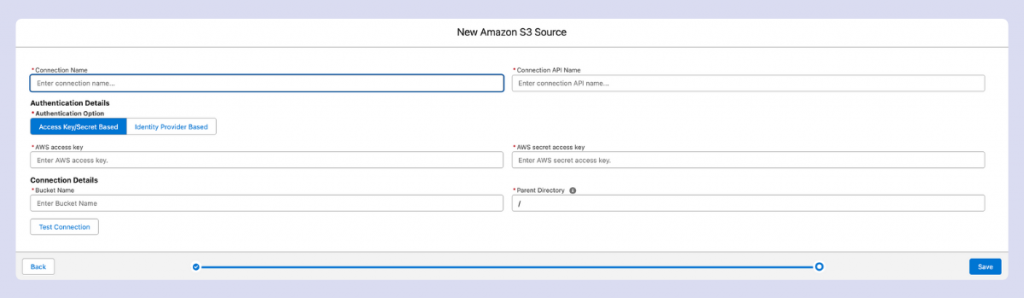
Note: When setting up an S3 connector, your configuration will be limited to a single bucket. If you need to access multiple buckets, you’ll need to create separate connector configurations.
Returning to the stream creation process, select your configured connector:
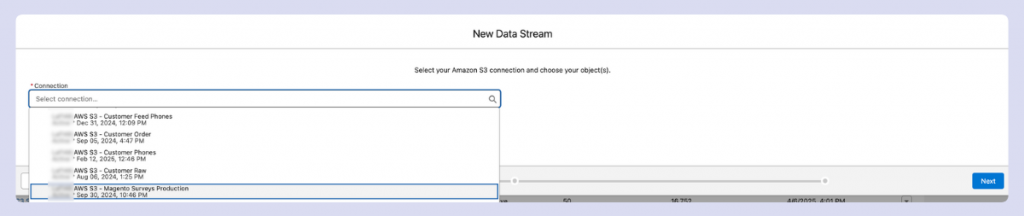
With your data source now accessible, let’s examine the core ingestion process in the next section.
The essentials of configuring your data stream
After selecting your connector, you’ll need to specify the data format. The two most common options are CSV and Parquet.
CSV is widely used and human-readable. However, Data Cloud currently offers limited options to customize CSV parsing details, which can sometimes lead to data issues.
Parquet, while not easily readable by humans, tends to be more error-resistant during Data Cloud processing, reducing the likelihood of column integrity problems.
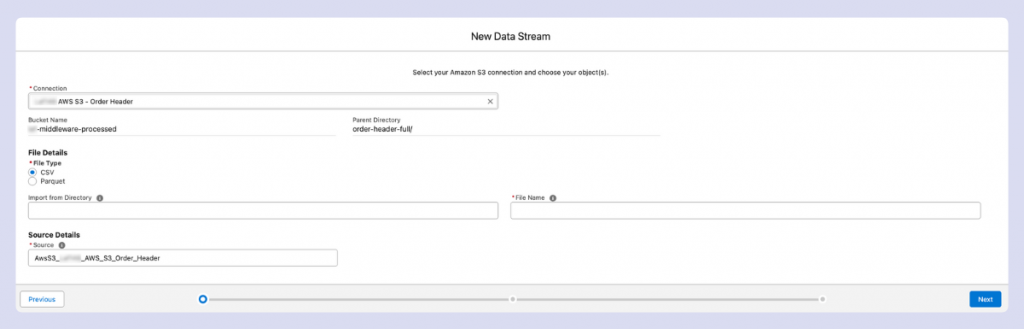
Next, specify the directory and file name for your data source.
Data Cloud supports the asterisk (*) wildcard character, which is a powerful feature. In data contexts, the asterisk represents “any character, any number of times.”
For example, if you have daily order files in an S3 bucket with names like “DailyOrderFeed-20042025.csv” and “DailyOrderFeed-21042025.csv,” you can use:
- DailyOrderFeed-*.csv
This pattern will capture all files that start with “DailyOrderFeed-” and end with “.csv”. You can also use just “*” as the filename to include all files in the specified directory.
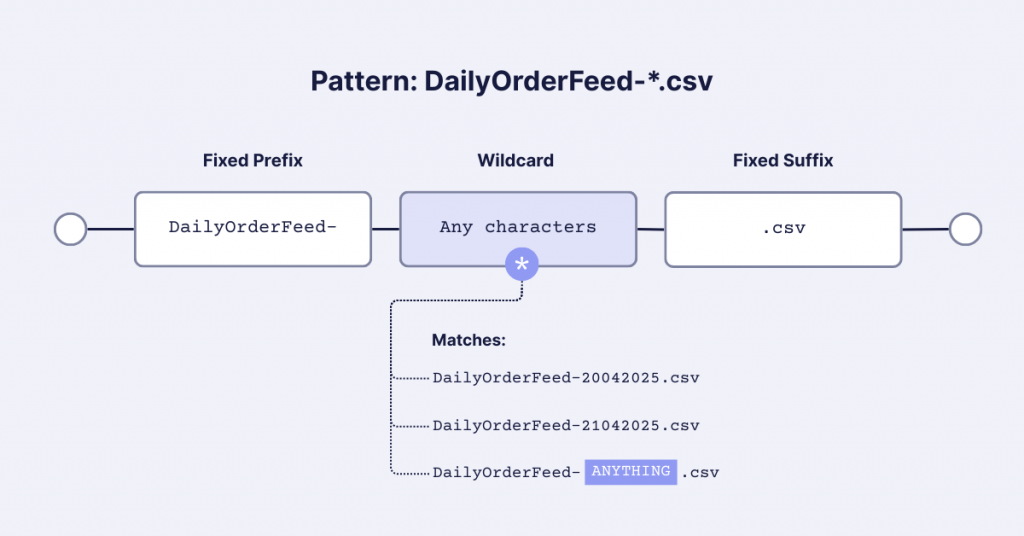
On the next screen, you’ll name your Data Lake object. The API name generates automatically but can be modified if needed.
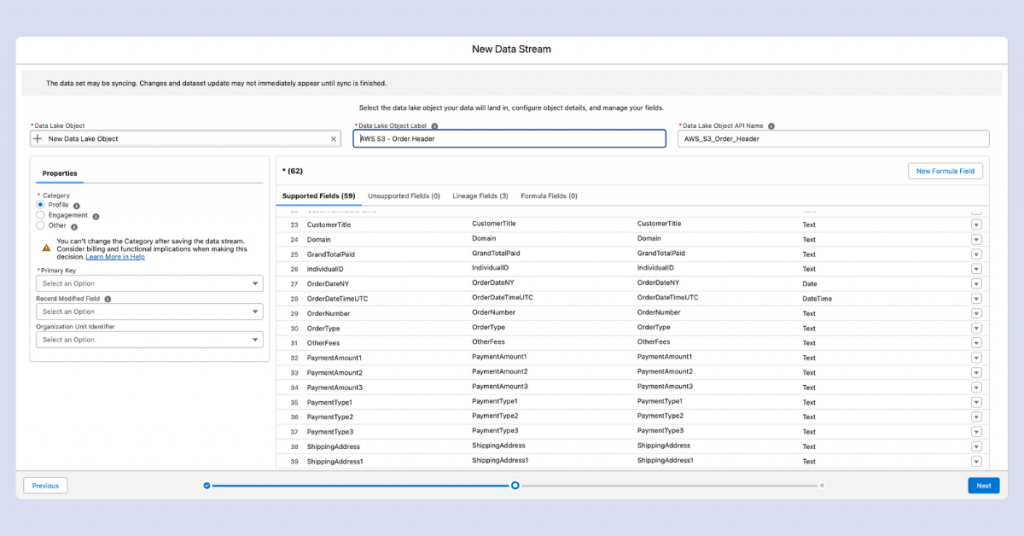
The category selection is critical and cannot be changed after creation:
- Profile – For customer attribute data (name, email, phone, country, etc.). Cannot map to Engagement DMOs.
- Engagement – For event data (purchases, email opens, website visits). Must include a date field. Cannot map to Profile DMOs.
- Other – The most flexible category for data that fits neither Profile nor Engagement. Can initially map to any DMO category, but once mapped to either Engagement or Profile DMO, cannot switch to the other type.
The primary key selection is crucial for data integrity. This field helps Data Cloud identify unique records. For example, using an order number as a primary key keeps only one record if two orders have the same number.
Remember: primary keys must be unique! If you choose a non-unique identifier, you risk losing records. For engagement events like email interactions, creating a composite key (combining values like customer email + event datetime + event name) can solve uniqueness issues.
The Record Modified field determines which version of duplicate records to keep. If two records share the same primary key, Data Cloud will retain the record with the more recent Record Modified timestamp.
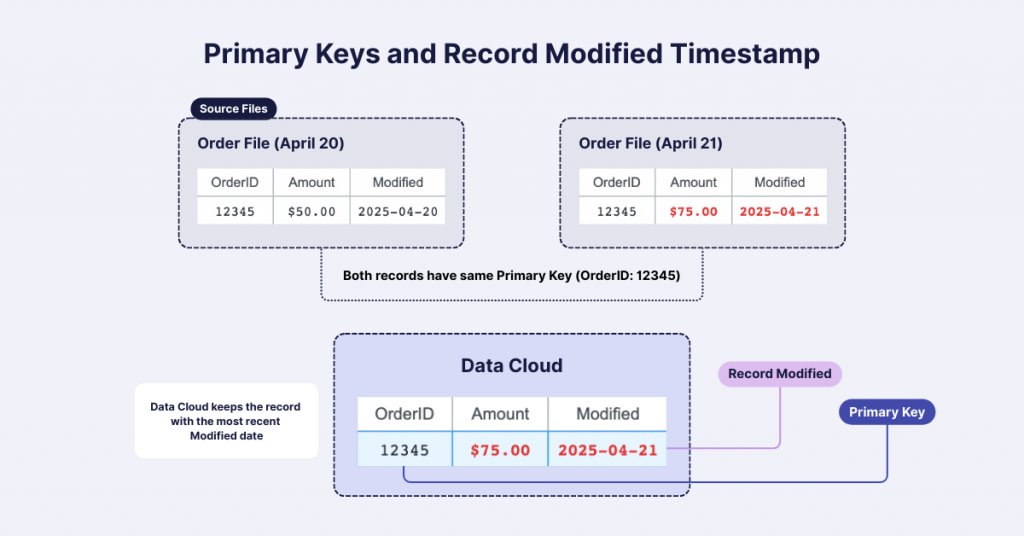
For Engagement streams, you must also define an Event Time Field.
Organization unit settings are only needed if you’re working with multiple organization units.
When defining data types for your fields, review each column carefully. Data Cloud attempts to determine types automatically, but it isn’t perfect—it might incorrectly classify ZIP codes as numbers when they should be text. You cannot modify column types after stream creation.
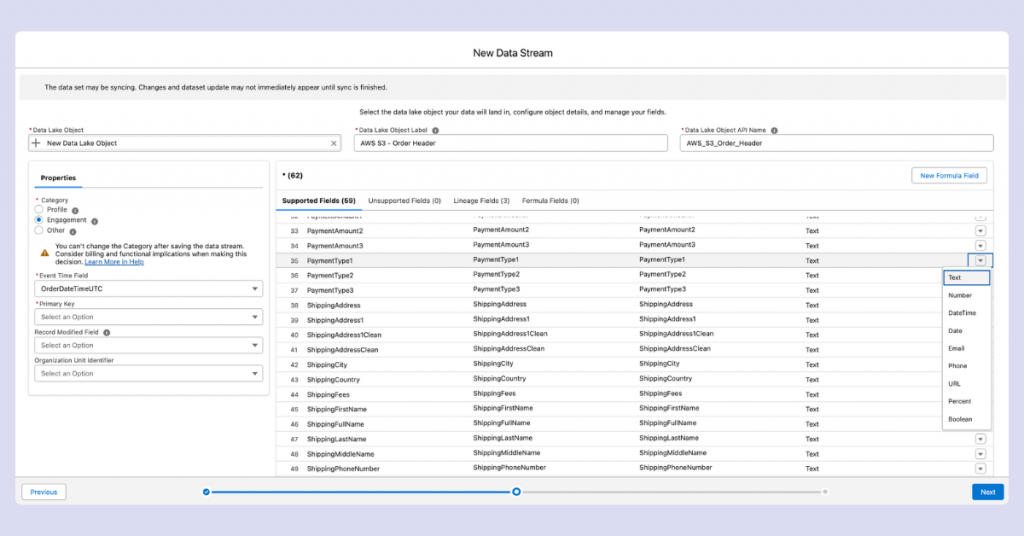
You can also create formula fields using supported functions. Common use cases include creating composite primary keys or, for example, combining first and last names into full names.
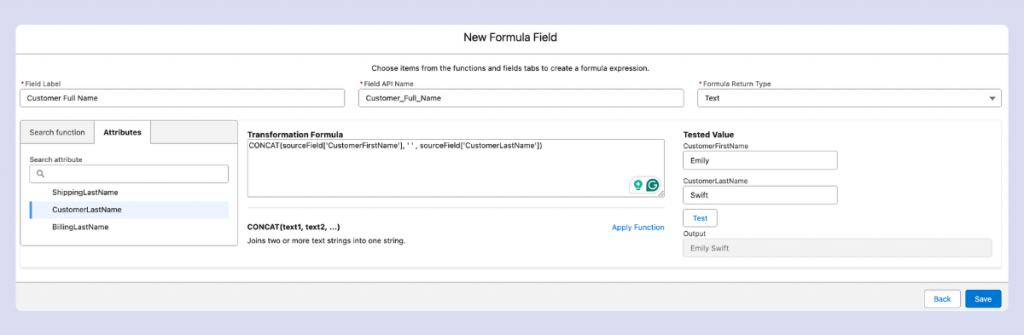
The final configuration screen appears before deployment:
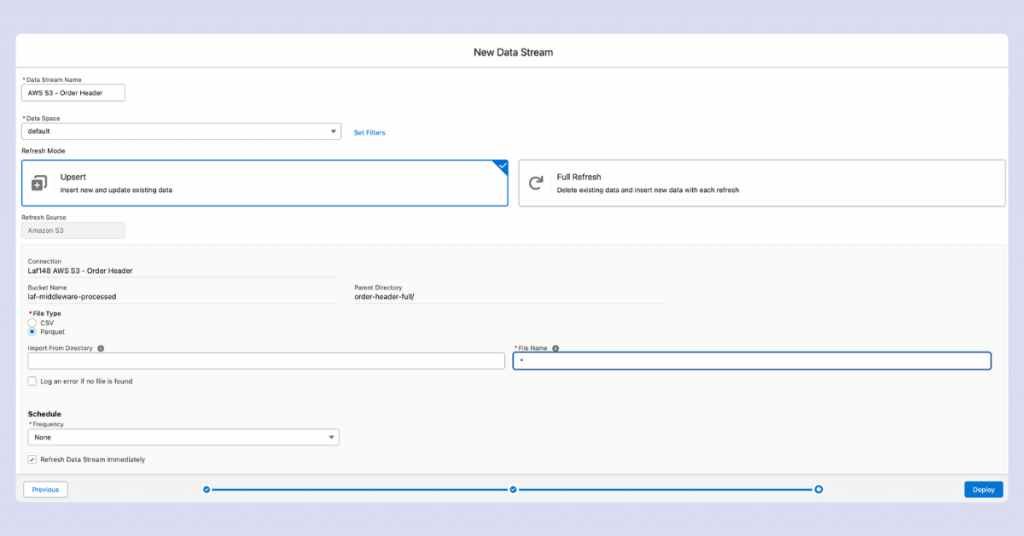
You’ll need to choose between two update modes:
- Upsert – Updates existing records if the primary key matches, or adds new ones if it doesn’t; old records stay, even if the source file disappears.
- Full Refresh – If records disappear from source files, they’ll also be removed from the stream.
You can modify refresh modes after stream creation.
Finally, set your update frequency schedule. Without this, your stream won’t update even if new files appear in the source location.
Once you’ve reviewed all settings, it’s time to bring your data stream to life. Hit the “Deploy” button to start moving your data into Salesforce Data Cloud!
How data cloud handles file updates and refreshes
When working with data streams in Data Cloud, we discovered an important behavior.
Data Cloud tracks which files it has already processed by their filenames. If you update the contents of a file without changing its name, Data Cloud may not detect or process these changes in either refresh mode.
This becomes particularly noticeable when you expect a Full Refresh to completely update your data. Despite selecting this option, if your updated source file retains the same filename as the previously processed version, your changes might not appear in the stream.
For reliable updates, consider implementing a filename versioning system (like adding timestamps or version numbers) to ensure Data Cloud recognizes and processes your updated files.
Updating your stream settings after deployment
You can adjust certain stream configurations after initial setup. Adding formula fields remains possible, but it’s important to understand that these changes only apply to new data going forward.
For instance, if you create a ‘Full Name’ formula field that combines ‘First Name’ and ‘Last Name’, this field will only appear in records processed after you added the formula. Later, if you modify this formula to also include ‘Middle Name’, that updated version will only apply to records processed from that point on.
When your source data evolves to include additional columns, Data Cloud allows you to incorporate these new fields into your stream. Click ‘Add Source Fields’, and Data Cloud will display any new fields it has detected in your source. You can also manually add columns through this interface if needed.
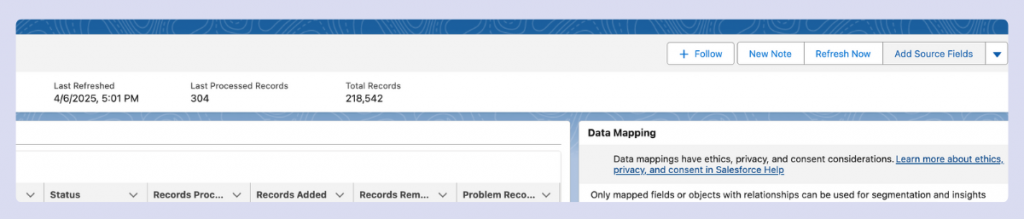
Problem records DLOs
You may notice DLOs with names starting with ‘PR_…’ in your Data Cloud environment. These are Problem Records—a special category created automatically when Data Cloud encounters issues processing certain records from your data streams.
Problem Records contain data that couldn’t be properly ingested due to various issues like data type mismatches, missing required fields, or formatting problems. Data Cloud separates these problematic records instead of failing the entire batch, allowing successful records to continue processing while isolating the problematic ones for your review.
Checking and validating your ingested data
After successful data ingestion, you’ll want to verify the data quality and ensure everything imported correctly. Data Cloud offers two main tools for this purpose.
The simplest approach is to use Data Explorer, accessible from the Data Cloud menu. This tool provides a quick preview of your data and supports basic filtering capabilities, making it ideal for initial data validation.
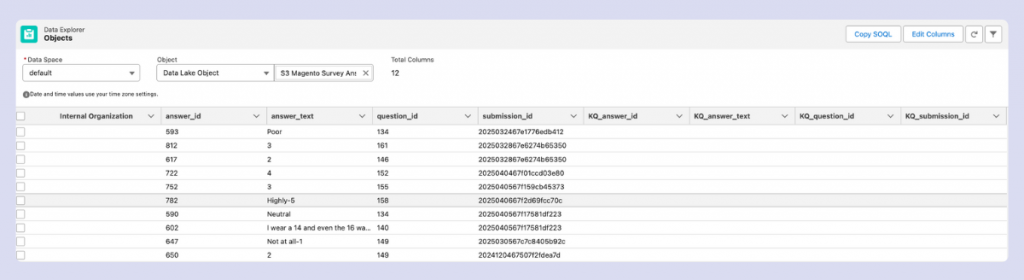
For more comprehensive data validation, the Query Editor offers advanced capabilities. Also found in the Data Cloud menu, this tool incorporates Data Explorer functionality but adds powerful SQL-based analysis options. Here you can create complex queries with aggregations, advanced functions, and sophisticated filtering to thoroughly examine your data integrity and quality.
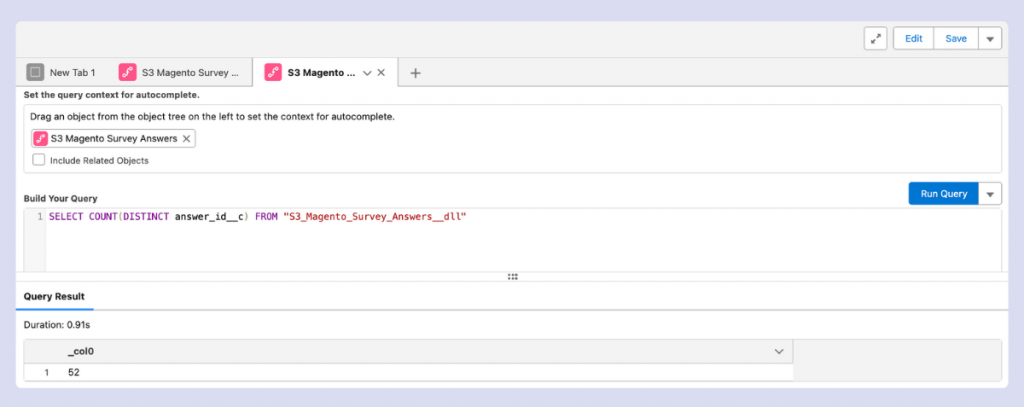
If your company has a strong data system already with data stored and transformed in cloud, it’s best to do the first quality checks there before sending data to Data Cloud. This helps catch and fix issues earlier in the data pipeline.
Still, even with clean source data, you should check how Data Cloud processes it. Data type conversions, formula fields, or connector-specific behaviors can sometimes alter data in unexpected ways. In these cases, Data Cloud’s native tools are ideal to test how your data is actually being processed within the platform.
The Query Editor is particularly valuable when you need to verify relationships between datasets, check for missing values, or validate business rules across your ingested data. Its SQL interface gives you the flexibility to construct exactly the verification checks your use case requires.
How to safely delete streams and data assets
When removing data assets from Data Cloud, understanding dependencies is essential. You cannot delete a DSO or DLO that has active connections to other components.
Streams mapped to data models must typically be unmapped before deletion. This becomes particularly challenging if the asset is used in identity resolution or calculated insights. Remember that dependencies flow downstream—a single DLO might feed into multiple models, segments, insights, and activation targets.
In certain situations, you can delete a mapped stream directly, specifically when the mapping exists but isn’t being used in any active downstream processes. However, this behavior isn’t consistent and shouldn’t be relied upon. In complex cases, you might need to delete an identity resolution ruleset first, then remap and reingest your data.
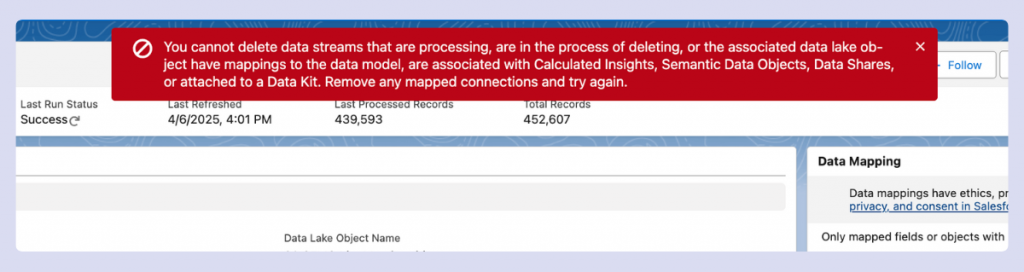
Plan your data architecture carefully from the start to avoid these complications. A clear understanding of your asset relationships will make future modifications much simpler.
Key takeaways
Setting up data ingestion in Salesforce Data Cloud takes careful planning and attention to detail. From choosing the right connectors and file formats to managing problem records and understanding dependencies, each step builds the foundation for reliable, scalable data operations.
Taking the time to get it right early will save you a lot of time, trouble, and costs down the road.
Need help setting up or optimizing your Salesforce Data Cloud? Our team is here to help. Get in touch to make your Data Cloud project a success.
Share on: